As global supply chains become more complex, businesses are seeking out innovative solutions to optimize their operations and stay ahead of the competition. One of those solutions is the use of predictive analytics in supply chain management.
So, how does predictive analytics play a role in it?
You’ll find out in this article. We’ll dive deeper into the world of predictive data analytics and demonstrate how it can change the way you manage supply chains. Additionally, we’ll give examples of how predictive analytics can be applied to specific industries that deal with supply chains.
There will also be case studies to showcase its successful implementation. By the end of this, you’ll have a greater understanding of its impact and how it can boost your bottom line by streamlining your operations and being prepared to tackle challenges.
Let’s explore the world of predictive analytics in supply chain management and see how impactful it is.
What Is Predictive Analytics And Why Is It Important In The Supply Chain Industry?
Predictive analytics is a type of advanced analytics that involves using statistical algorithms and machine learning techniques to analyze historical and current data to predict future events or behaviors. In the fast-paced business environment, it has become a valuable tool in many industries, including the supply chain industry.

In this industry, predictive data analytics can help supply chain companies make better decisions, which can optimize your business processes. For instance, you can collect quality data from sources like customer feedback, sales, inventory levels, weather forecasts, and transportation data.
With it, you can anticipate demand, optimize the inventory management process, and reduce costs. This is beneficial to minimize risks and losses.
Implementing predictive analytics models in supply chain companies or departments can be challenging. It requires significant investment in big data collection and analysis infrastructure.
Plus, you’ll need a deep understanding of business processes and customer needs. You’ll also need to learn its different techniques on how it can be done like regression analysis, neural networks, text analytics, and decision trees.
However, it’s worth it. With access to as much relevant data and market trends, you can make better-informed decisions, improve operational efficiency, and increase profitability.
Following this, it’s time to get into the core of predictive analytics and see how it all works in the industry.
How Does Predictive Analytics Work In Supply Chain?
Predictive analytics in supply chain processes helps to anticipate future trends, identify potential risks and opportunities, and manage big data more efficiently. This aids supply chain professionals to optimize the overall supply chain operations.
To further illustrate the impact of predictive analysis, let’s get some practical use examples.
1. Demand Forecasting
Precise prediction of demand is crucial in supply chain management. It satisfies customer demand while minimizing inventory expenses.
Historical data is a key component when it comes to forecasting demand. By looking at past trends, companies can get an idea of what kind of products or services will be in high demand in the future.
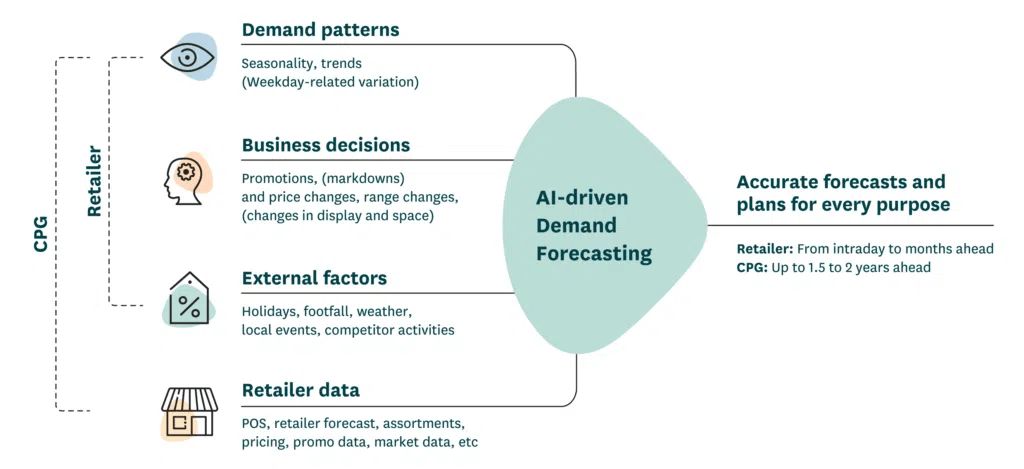
In addition, you could use data management techniques to collect and store data on sales, promotions, weather patterns, and other factors that affect demand. Through data analysis, companies can identify patterns and trends that can help them in making more precise predictions of future demand.
Predictive models take factors like seasonality, economic indicators, and consumer behavior into account to predict demand for each product or service.
1.1 Example: Supermarket Chain
It can leverage predictive models to predict the demand for particular products by utilizing past sales data and trends in the market.
For instance, if the data shows that customers usually buy more cereal during winter, the store can order more stock of the new cereal in the fall to prepare for the increase in demand during winter.
In doing so, its supply chain can optimize inventory and reduce the risk of overstocking or understocking. Hence, satisfying customer demand without incurring losses.
1.2 Example: Retailer
A clothing retailer can leverage predictive analytics to get the demand forecast for its products through the analysis of historical sales data, social media trends, and weather patterns. By analyzing those factors, the retailer can gain a comprehensive understanding of consumer behavior, social media sentiment, and even seasonal trends.
For instance, if the data shows that summer is about to begin in a specific country, the retailer can order more stock of those items in advance for their shops in that region. Thus, ensuring that it has enough inventory to meet consumer demand.
Demand forecasting is a critical aspect of supply chain management, and data analytics and predictive solutions can help companies forecast demand accurately. The examples highlight how predictive analysis can help forecast customer demand and trigger actions in the supply chain.
2. Logistics/Transportation Optimization
Logistics is crucial as it manages the goods of a company from origin to consumption. It ensures that the right product is delivered to the right place, at the right time, and in the utmost condition. It plays a critical role in improving overall supply chain performance.

Predictive data analytics in logistics have a big impact on supply chain management and overall company success. Companies can optimize their transportation networks by analyzing historical shipping data, traffic patterns, weather conditions, and other relevant factors to identify the most efficient routes and modes of transportation.
It results in increased customer satisfaction, better supplier relationships, and reduced costs.
Predictive analytics models can also help you in risk management for your logistics functions. By utilizing advanced data analysis techniques, you can identify potential issues and bottlenecks in the supply chain and proactively mitigate risks to prevent disruptions.
This includes predicting supplier delays, natural disasters, and transportation issues, among others. Also, predictive analytics in the supply chain helps maximize operational efficiency.
By examining big data analytics, you can improve your logistics planning and make informed decisions. As such, you can optimize fleet performance and improve route planning among other things.
You don’t need to look far to get all of that. DiLytics has dabbled in providing solutions for logistics and supply chain companies to help address challenges by leveraging analytics. By implementing our predictive analytics solution, you can improve your decision-making and identify revenue leakage among other things.
2.1 Example: Food Industry
Food companies need to ensure that their products are transported quickly and efficiently to maintain freshness and quality. Predictive analytics models can help supply chain managers optimize fleet performance by analyzing data on shipping routes, weather patterns, and traffic patterns.
Moreover, predictive analytics tools can help determine the most efficient transportation routes and modes of transportation to reduce costs and improve delivery times. The use of predictive data can also accurately predict the transportation needs of perishable goods. Hence, ensuring that the goods are delivered on time and remain fresh.
2.2 Example: Pharmaceutical Industry
Companies in this industry face strict regulations and requirements for transportation to ensure the safety and efficacy of their products. For example, a pharmaceutical company may use predictive models to optimize the transportation of temperature-sensitive medications.
Scrutinizing historical data on shipping routes, weather patterns, and other relevant factors can help the company reduce transportation costs, improve delivery times, and ensure the safety and efficacy of its products.
Overall, the use of predictive analytics can help companies, especially the ones dealing with time-sensitive products, identify the most efficient routes and modes of transportation. This helps logistics companies or departments to streamline operations and reduce risks.
With reliable data and predictive analytics solutions, your supply chain leaders can have better decision-making capabilities.
3. Supply Chain Risk Management
Predictive analytics models can identify patterns and trends that can help you anticipate and mitigate risks before they occur. The analysis of current and historical data can help you better understand your supply chain, identify potential risks, and take proactive steps to reduce exposure to those risks.
What’s more, predictive analytics solutions can also help you identify important supply chain metrics that can be used to track performance and identify areas for improvement. For example, by analyzing big data, you can identify trends in delivery times, inventory levels, or production output. The image below shows more factors you need to consider.

Identifying key metrics and tracking them over time allows you to pinpoint the areas where you need to focus your risk management efforts. For example, one key area where predictive models can be applied is predictive maintenance.
It can help identify potential equipment failures before they occur by analyzing data from sensors and other sources. As such, you can schedule maintenance at optimal times, minimizing downtime and reducing the risk of costly repairs.
3.1 Example: Manufacturing Industry
To keep production plans on track and meet consumer demand, manufacturing companies ensure a steady stock of raw materials and supplies. Fortunately, you can adopt predictive insights to reduce supply chain risks with the aid of predictive analytics.
Collecting data from suppliers, transportation routes, and logistics providers, and utilizing machine learning algorithms and statistical methods help you to identify potential disruptions and take proactive measures to minimize the impact.
For example, a manufacturing company may use predictive models to analyze big data on supplier performance, including lead times, quality metrics, and delivery schedules. Another example is to analyze data on machine performance, production rates, and maintenance schedules to optimize production output and reduce downtime.
3.2 Example: Retail Industry
Retail companies can collect actual data on various aspects of their supply chain, such as transportation routes, lead times, inventory levels, weather patterns, and consumer demand. This data can be used to create models that predict future outcomes and recognize possible risks.
For example, a retailer might analyze historical data on weather patterns and transportation routes to identify areas that are likely to experience delays or disruptions during certain times of the year.
By doing so, the company can take proactive measures to minimize the impact of these disruptions, such as sourcing products from alternative suppliers or using alternative transportation routes.
Using predictive solutions like DiLytics can minimize the risk and help you overcome challenges in the supply chain. As such, you can be proactive instead of reactive. You can maintain product availability, minimize losses, and improve the overall supply chain performance.
Now that we know how predictive analytics in the supply chain works, it’s time to take on some real-life examples. Let’s see how implementing predictive analytics has helped companies determine prospective trends.
Supply Chain Predictive Analytics: How Predictive Analytics Capabilities Guide Companies
Implementing predictive analytics in the supply chain is a cutting-edge approach to operations management. It leverages data storage, data mining, and machine learning algorithms to guide companies, like yours, in making informed decisions about the supply chain processes.
To implement predictive analytics, you should start by collecting and analyzing historical data. Then, use predictive analytics solutions to make demand forecasts and identify patterns that inform the decision-making process.
Have a look at the case studies below to get a greater understanding.
Case Study 1: Biotechnology Company
The client was an American biotechnology company. Lack of access to supply chain planning data meant that the company didn’t have visibility into the latest information. As a result, their planners had to invest significant manual effort to obtain up-to-date data to create reports.

They experienced obstacles and delays in responding to common ad hoc questions, which were necessary to support management decisions. Fortunately, a predictive analytics solution came to the rescue.
The Solution: DiLytics’ Supply Chain Planning Analytics
This situation improved with the implementation of predictive analytics in supply chain planning. With better access to real-time and historical data, the company’s planners reduced manual effort and improved forecasting capabilities leading to better decision-making.
The utilization of predictive models allowed the company to have insights into:
- An order forecast
- Inventory overview and balance
- Safety stock compared to projected inventory
- Comparison between planned and actual quantities of shipped products
By implementing predictive analytics solutions, the company streamlined its processes and quickly answered ad hoc questions, reducing delays and improving overall efficiency. DiLytics’ approach saved the company’s time and resources while improving the accuracy and reliability of supply chain planning data.
Case Study 2: Container Leasing Company
A top container leasing company initiated a Business Intelligence Program to fulfill its analytical reporting requirements while reducing its reliance on IT. Its need isn’t exactly to implement predictive analytics, but the integration of the custom solution does affect the supply chain.
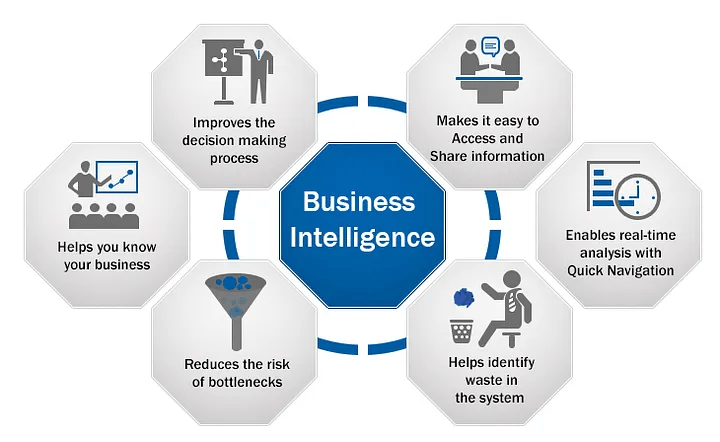
The Solution: DiLytics’ Custom Analytics
DiLytics installed an enterprise data warehouse solution that gathered data from various in-house systems and satisfied the reporting demands of the different departments and functions of the whole company.
Additionally, the use of the custom analytics solution allowed the company to streamline its decision-making processes and optimize its business operations. Although, this initiative could be further improved by implementing predictive analytics models.
By integrating it into its enterprise resource planning, the company can leverage data to forecast future trends and gain insights, ultimately improving its bottom line.
C. Case Study Number 3: IBM
IBM, the owner of The Weather Company, uses Watson to make accurate weather predictions, which impacts consumer behavior. By analyzing data from multiple real-time sources, such as social media and news stories, IBM can identify how customers react to weather conditions and make informed decisions on the supply chain.
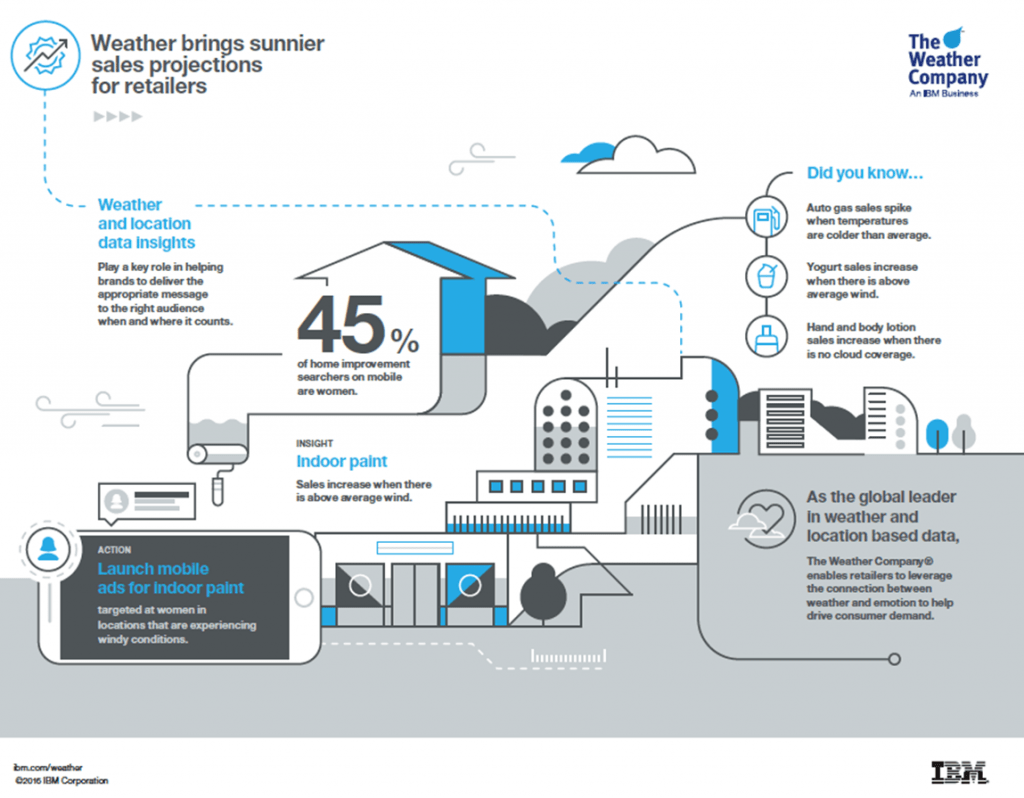
This approach allows the company to leverage the commercial opportunities that arise from weather conditions. Additionally, IBM’s technology allows retail companies to integrate this information with their existing data, enabling demand planners to create more accurate demand plans and sales and marketing teams to promote and price products accordingly.
With that, let’s jump into another type of data analytics that’s often used with the predictive method.
Want to know how Predictive Analytics can help you strengthen your supply chain processes and make data-driven decisions? Simply fill out the form below. Share some basic information, and our team will promptly reach out to you.
Prescriptive Analytics: Its Role & Impact On Your Business
Prescriptive analytics is a type of analytics that uses data collected from diverse sources, such as big data, to recommend the best course of action for a given scenario. It utilizes optimization techniques such as a mathematical model, machine learning, and artificial intelligence to deliver actionable insights.
What’s more, it plays a critical role in predictive analytics by taking the predictions generated by the predictive analysis and providing recommendations for action based on those projections of future events or trends.
In regards to the supply chain networks, here’s how prescriptive analytics working with predictive analysis can guide companies:
- Fuel consumption optimization: It can help supply chain managers identify the most efficient routes for transportation and minimize fuel consumption.
- Supplier selection: It can assist managers in selecting the best suppliers or supply chain partners by analyzing various factors such as quality, lead time, and cost.
- Production optimization: By recognizing bottlenecks and offering recommendations, prescriptive analysis can help managers optimize production schedules through improved workflow and productivity.
- Inventory management optimization: It helps managers keep track of the demand and make sure that the stocks are always at optimal levels. Thanks to demand forecasts, it’ll ensure the company has enough stock to meet customer demands while avoiding overstocking or understocking.
Overall, it helps heighten the impact of predictive analytics too. As such, you’ll know how to maximize the results you get from predictive models. It can guide you to make better decisions based on data-driven insights.
Conclusion
The role of predictive analytics in supply chain management is crucial. Its diversity for different industries and types of businesses has helped many entrepreneurs to drive growth for their companies.
So, are you ready to follow their path and take the leap?
Well, here at DiLytics, we can take care of you. Whether you have a supply chain company or just want to improve the supply chain function of your business. We have a specialized solution or you can implement custom analytics depending on your business needs. Learn more about us and check out how we can help you.